At first glance, the idea that Nvidia could be in a strategic dilemma seems far-fetched. Not when its stock just exploded by over $200 billion in a single day to set a new market record. Jensen Huang is on top of the world right now as CEO, arms stretched to the heavens receiving the riches and plaudits raining down on his company.
To uncover Nvidia's strategic problem is to understand how technology and competition evolves along long waves of innovation. This is not a discovery I made myself, it is a key insight I learned from Simon Wardley. For reasons I do not fully understand, Simon Wardley decided to just give away some of the best thinking on technology strategy in the last 30 years, building on the ideas of Everett Rogers.
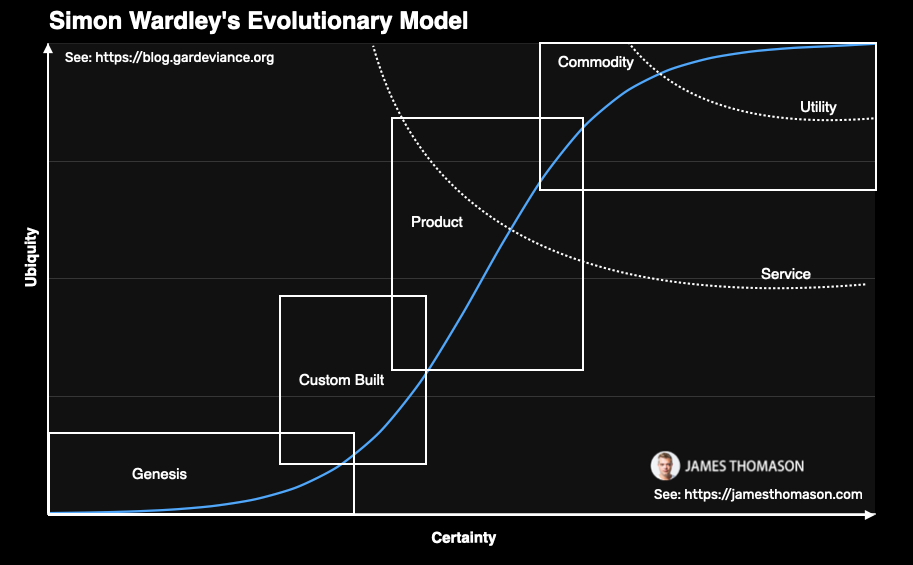
As Everett Rogers noted, the long wave is composed of many shorter technological waves, each with their own diffusion process of "crossing the chasm". One of Simon Wardley's breakthroughs was the realization that technologies are not simply created and diffused to ubiquity, they evolve, as does competition.
This evolution is predictable, from Genesis, to Custom Built, Product, and Commodity or Utility:
Genesis: This is what we think of as pure Research and Development, it is the development of a brand new technology the world has never seen. We are not very certain of its value, of how to build it, productize it, or take it to market.
Custom Built: Most technologies begin their commercial life as a large, clunky, expensive product where prices are high and relatively few units are sold. This is often necessary to instantiate the supply chain and can take a long time.
Product: We think of a product as a technology that is mass produced in the many thousands to tens of millions of units per year. Supply chains benefit from increased standardization and commoditization resulting in lower unit prices.
Utility: This is the point at which the customer is no longer asked to bear the capital cost or operations and maintenance of the product. The product is priced as a unit of value or consumption. The supply chain is very mature and efficient reducing margins and prices along the chain.
The more ubiquitous a technology becomes, the greater the effect of commoditization and componentization along the supply chain, culminating in the creation of services and ultimately utility models.
The situation
I believe NVIDIA is at the forefront of the next long wave of innovation that will transform industry for the next 25-50 years. The question is: how long can Nvidia ride this wave before it crashes into the shore?
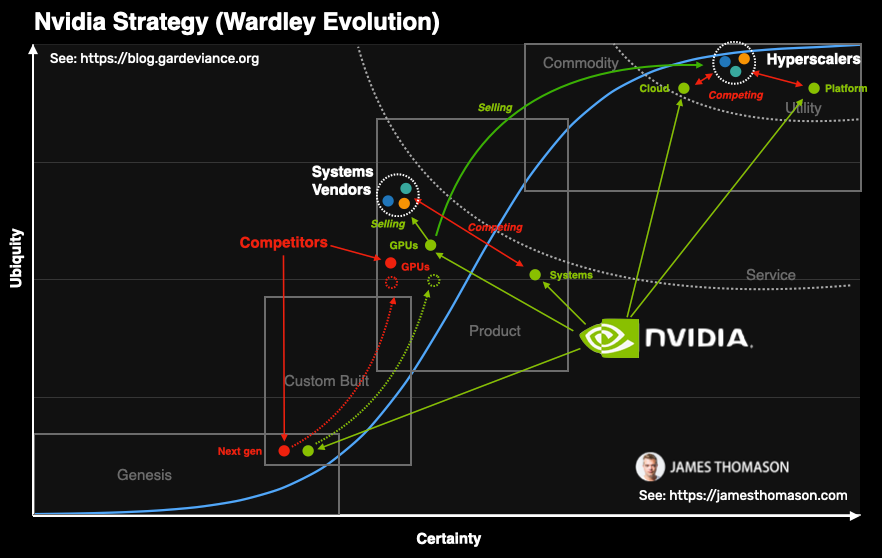
NVIDIA finds itself navigating a complex strategic position between collaboration and competition within its own ecosystem:
Systems Vendors: As a premier manufacturer of GPUs, NVIDIA has long been a crucial supplier to systems vendors like Dell, HPE, Cisco, and Supermicro. This relationship, however, is beginning to show signs of strain as Nvidia ventures into the realm of complete system solutions, pitting itself against its own customers in a race for GPU allocation.
Hyperscalers: The scenario grows even more difficult when considering the hyperscale cloud market—a domain where NVIDIA's GPUs are in high demand for their unparalleled processing power essential for AI and deep learning tasks. NVIDIA's foray into this space with its cloud offerings and NVIDIA AI Enterprise platform escalates the tension. By providing a comprehensive suite designed to streamline workload orchestration, NVIDIA positions itself as a direct rival to the giants of cloud computing—Amazon, Microsoft, and Google. These entities, keen on locking developers into their ecosystems through proprietary tools and services, will view NVIDIA's encroachment with caution, as it threatens to disrupt their control over the developer value chain. Hyperscalers are also increasingly developing and deploying their own custom chips. This move represents a strategic pivot away from reliance on traditional chip manufacturers, including NVIDIA, to fulfill their hardware needs. By designing chips in-house, these behemoths aim to tailor performance to their specific applications, such as AI workloads, data center operations, and consumer electronics, achieving greater efficiency and optimization than generic solutions can offer.
Direct Competitors: Then, there is the looming threat of direct competitors like AMD and Intel. These companies will aggressively develop alternatives, aiming to undercut NVIDIA on price and erode its market share. This introduces a significant challenge as NVIDIA must navigate the delicate balance of maintaining its innovation lead and market dominance while contending with the inevitable price and margin pressures these alternatives will bring.
Technological Threats: The development of more efficient learning and inference algorithms represents a double-edged sword for NVIDIA. As these algorithms evolve, they require less brute-force computational power, potentially reducing the reliance on NVIDIA's high-powered and extremely expensive GPUs. This evolution is at the heart of the artificial intelligence sector's progress, making AI models more accessible and less dependent on specialized hardware.
A historical perspective
The semiconductor industry is characterized by rapid innovation, short product life cycles, and shifting competitive advantages. The duration for which a chip manufacturer can maintain its competitive edge varies widely and depends on several factors including technological advancements, market demand, strategic partnerships, and investment in research and development. The history of semiconductors shows us that dominance in this sector can last anywhere from a few years to a couple of decades, with the transition periods often marked by significant technological breakthroughs or shifts in market needs.
IBM and Motorola: In the early days of the semiconductor industry, companies like IBM and Motorola were pioneers, leading in various segments from the 1960s to the early 1980s. Their dominance was particularly notable in areas like mainframe computers and early microprocessors. However, as the market evolved towards personal computing, their leadership waned.
Intel: Intel's dominance began in the late 1980s and continued through the 1990s into the 2000s, driven by its success in personal computer microprocessors. Intel's competitive edge lasted for several decades, making it one of the most enduring leaders in the semiconductor industry. The "Intel Inside" marketing campaign and its strategic partnerships with computer manufacturers cemented its place in the PC market.
AMD: AMD has been a significant player since the 1980s but often as an underdog to Intel. However, it started to capture a more substantial market share in the early 2000s and then again more recently in the late 2010s, thanks to its advancements in CPU and GPU technologies. AMD's competitive cycles have fluctuated more compared to Intel but have shown remarkable resilience and innovation.
NVIDIA: NVIDIA's rise to prominence began in the late 1990s with the success of its graphics processing units (GPUs) for gaming. However, NVIDIA's significant competitive edge was established in the mid-to-late 2010s, with its GPUs being highly suited for AI and deep learning applications, a market that has seen explosive growth.
ARM Holdings: ARM's influence in the semiconductor industry underscores a different kind of dominance, rooted in intellectual property and architecture design rather than manufacturing prowess. Since its founding in the early 1990s, ARM has become the leading architecture in mobile computing, with its designs found in billions of devices worldwide. Unlike companies that manufacture their chips, ARM licenses its designs to others, including Apple, Qualcomm, and Samsung, who then produce ARM-based processors. This model has allowed ARM to become ubiquitous across a wide range of computing devices, especially in the mobile and low-power device markets. ARM's dominance is characterized by its widespread adoption and the critical role its architectures play in enabling mobile technology and, increasingly, areas like autonomous vehicles and edge computing.
Others: Many other companies have had periods of dominance or significant influence in various niches of the semiconductor market. For example, Qualcomm has been a leader in mobile processors, and Texas Instruments in analog semiconductors and digital signal processors. South Korean giant Samsung Electronics has also been a significant player in memory chips and is a leading manufacturer of NAND flash memory and DRAM.
The cycles of dominance in the semiconductor industry, which can be roughly estimated to last from 10 to 20 years, have historically aligned with major technological shifts such as the move from mainframes to PCs, the rise of mobile computing, and the current surge in AI and machine learning. However, this time might be different due to the self-reinforcing nature of AI technology.
AI technologies are being used to design more advanced semiconductor chips, which in turn enhance AI's capabilities. This creates a powerful feedback loop: improvements in AI lead to better chip designs, which then further accelerate AI advancements. This self-reinforcing cycle has the potential to significantly compress the traditional cycles of dominance in the semiconductor industry or enable companies to maintain their leadership positions for longer periods by leveraging AI to drive innovation at an unprecedented rate.
AI winter
The history of artificial intelligence (AI) is punctuated by cycles of extreme optimism followed by disappointment and reduced funding, often referred to as "AI winters." Historically, these cycles in AI research and development begin with inflated expectations and investment in AI technologies, followed by a violent correction or a significant slowdown.
First AI Boom: Late 1950s to the mid-1970s
The first AI boom began in the late 1950s and early 1960s, a period characterized by initial optimism about the potential for machines to replicate human intelligence within a generation. This era saw significant investments in AI research, particularly in symbolic AI and problem-solving systems. However, by the mid-1970s, it became apparent that many of AI's early promises were far from being realized, leading to the first AI winter. This period of reduced funding and interest lasted into the early 1980s and was marked by skepticism towards AI's feasibility and practicality.
Second AI Boom: Early 1980s to the late 1980s
The second boom emerged with the rise of expert systems in the early 1980s. Expert systems were AI programs that simulated the knowledge and analytical skills of human experts, and they saw commercial success in various industries. This success led to a renewed surge in interest and investment in AI. However, by the late 1980s, the limitations of expert systems—such as their inability to generalize beyond narrow domains—became evident. The resulting disillusionment contributed to the onset of the second AI winter, which lasted into the early 1990s.
Third AI Boom: Early 2010s to Present
Some argue that a third AI boom began in the early 2010s, driven by advances in machine learning, particularly deep learning. These technologies have led to significant breakthroughs in areas such as image and speech recognition, natural language processing, and autonomous vehicles. The current period is characterized by substantial investment from both the public and private sectors, soaring valuations of AI startups, and heightened media attention.
AI Winter Is Almost Certainly Not Coming
Many people are publicly proclaiming the present moment as another "AI bubble". I think they are sorely mistaken. While there are concerns about overhyped capabilities and potential ethical and societal implications, the ongoing advancements in AI technologies suggest that the impact of AI is profound and long-lasting. The previous boom and bust cycles in AI were stalled because they were trying to do what was impossible using then-current technologies. This AI trend is much more indicative of the beginning of a new long wave of innovation, wherein many technologies will genesis, evolve, then deprecate in rapid succession as adoption increases.
What if deep learning is a dead end?
The human brain, with its nearly 100 billion neurons and up to 15,000 synapses per neuron, operates on an energy budget of about 20 watts, a stark contrast to the power-hungry nature of today's leading AI models which can consume over 300kwh.
This disparity brings to the possibility that deep learning, in its current form, might be approaching a technological barrier. It is hard to see how AI can become truly ubiquitous without major breakthroughs in computation and energy efficiency.
This looming threat suggests an eventual pivot towards a new computing architecture, one that draws inspiration directly from the brain's efficiency and complexity. For NVIDIA, the company's future growth and its position in the tech ecosystem could depend on its ability to anticipate and adapt to this shift.
The pursuit of brain-inspired computing architectures promises a new frontier for AI development, one that could overcome the limitations of current deep learning models. This approach aims to replicate the brain's energy efficiency and adaptability, potentially unlocking new levels of AI performance and application that are currently unimaginable. For NVIDIA, investing in research and development towards these next-generation technologies may be crucial.
NVIDIA could transition from merely supplying the horsepower for AI's current demands to leading the charge in a fundamentally new direction for AI technology. Such a shift would not only reaffirm NVIDIA's commitment to innovation but also position it at the forefront of the next wave of AI advancements. As the industry grapples with the limitations of deep learning, the move toward brain-inspired computing architectures could mark the dawn of a new era in artificial intelligence, redefining what's possible in the field.
Redefining its future beyond hardware
NVIDIA's current rise is fueled by an intense demand for its GPUs, integrated systems, and other hardware essential for AI, gaming, and data centers. For now, Nvidia's growth prospects look solid, supported by the market's robust demand.
However, this success is shadowed by the finite capacity to produce and sell physical products. Given the static margins of hardware, NVIDIA's growth is inevitably capped. The primary challenge is maintaining this momentum. As competitors introduce alternatives, Nvidia will face increased pressure.
In pursuit of expansion, Nvidia will continue to attempt new services and utility models. This shift, while strategic, brings Nvidia into direct competition with its biggest buyers: the hyperscale cloud giants like Amazon, Microsoft, and Google. These entities not only have vast resources but also a technological edge in scaling computing services, presenting a formidable challenge.
I think these attempts are not likely to succeed, and Nvidia could find itself relegated to its traditional position as a supplier. In that sense, Nvidia Enterprise AI could be a strategic mistake. Rather than attempting to monetize, Nvidia might be better off strategically if it took a page from the Google playbook and released everything as open source.
By releasing its technologies as open source, Nvidia could encouraging a broader base of developers and companies to build on its platforms. This approach not only undermines the control exerted by hyperscalers but also fosters a more vibrant ecosystem around NVIDIA's technologies, enhancing its influence and relevance in the tech landscape.